A collaborative study by Elliptic, MIT-IBM Watson AI Lab, and researchers using the Elliptic2 Data set, identified significant Bitcoin transactions linked to illegal activities such as money laundering. The study employed advanced machine learning techniques to analyze subgraphs within Bitcoin’s blockchain, distinguishing between legitimate and illicit transactions and improving the detection of criminal financial activities.
Key Facts
- The Elliptic2 Dataset contains 122,000 labelled subgraphs and is part of a larger dataset with 49 million node clusters and 196 million edge transactions.
- The dataset was used to identify Bitcoin transactions potentially linked to illegal activities like money laundering.
- Researchers used graph convolutional neural networks (GCNs) and experimented with subgraph classification methods, including GNN-Seg, Sub2Vec, and GLASS.
- The study successfully traced funds associated with suspicious transactions to a cryptocurrency mixer, a Ponzi scheme in Panama, and a Russian dark net forum.
- Recognized laundering methods include peeling chains and nested services.
- Future research aims to refine machine learning models and extend studies to other blockchain networks.
- Elliptic’s new approach involves collecting patterns of transactions from illicit entities to exchanges to train an AI model to detect similar patterns.
- The training data set released by Elliptic on Kaggle is the largest of its kind and is anonymized to protect privacy.
A recent analysis of a graph dataset that had Bitcoin transactions has recently found a large amount linked to illegal activities and money laundering. Elliptic and researchers from MIT-IBM Watson AI Lab conducted this analysis, which ended up showing key insights into criminal activities in the cryptocurrency space.
The Elliptic2 dataset is a large collection of graphs which is around 26 GB and contains 122,000 labeled subgraphs which represent the Bitcoin clusters. The clusters that were mentioned are part of a much larger graph that includes a huge 49 million node clusters and 196 million edge transactions. Elliptic2 is also known as an extension of the Elliptic Data Set (Elliptic1), which was released back in July 2019. The purpose of these datasets is to fight against this financial crime using graph convolutional neural networks (GCNs).
Tom Robinson: “Employing machine learning at the subgraph level can more accurately forecast whether the cryptocurrency transactions involve illegal financial gains.”
The analysis aimed to show the illegal activities and money laundering crimes by using the anonymity and the darker side of the blockchain. It joined this with information about both legitimate (like exchanges, wallet providers, and miners) and illegitimate services (such as darknet markets, malware, terrorist organizations, and Ponzi schemes) operating on the network.
Tom Robinson who is the chief scientist and co-founder of Elliptic, stated that employing machine learning at the subgraph level, which is made up of groups of transactions that are similar to money laundering, can more accurately forecast whether the cryptocurrency transactions involve illegal financial gains. This is quite different from the traditional anti-money laundering (AML) methods in the cryptocurrency space, which usually track funds from known illicit wallets or match patterns with recognized money laundering practices.
In their study, the researchers eventually experimented with three different subgraph classification methods on the Elliptic2 dataset: GNN-Seg, Sub2Vec, and GLASS. These methods were used to try and identify the subgraphs that were representing the cryptocurrency exchange accounts that were potentially involved in illegal activities.
Making Moves in Tracing and Understanding Cryptocurrency Laundering Patterns
The study also had a win. They successfully traced the main source of funds that were tied with the suspicious subgraphs to systems that included a cryptocurrency mixer, a Panama-based Ponzi scheme, and an invite-only Russian dark net forum. This research shows the real effectiveness of machine learning and graph analysis in finding and tracking illegal activities in the cryptocurrency space, also giving very valuable insights for fighting against financial crimes in the digital asset space.
One big discovery from the study was the recognition of well-known cryptocurrency laundering methods, including peeling chains and nested services. A peeling chain is a technique where a small amount of cryptocurrency is sent to one address while the rest is sent to another address and is completely controlled by the user. Although this method can be used for more legitimate financial privacy reasons, it can also be a way for more money laundering to take place, particularly when the “peeled” cryptocurrency is continually directed to a crypto exchange service. The importance of finding as well as understanding these patterns in detecting and bringing a stop to illegal financial activities within the cryptocurrency ecosystem is becoming more and more needed in this day and age.
Stefan Savage: “This is on the edge where people get uncomfortable in the same way they get uncomfortable about face recognition. You can’t quite explain how it works, and now you’re depending on it for decisions that may have an impact on people’s liberty.”
The research that is always ongoing will hold its focus on increasing the accuracy of the techniques that were used in this study. This also includes the further process of perfecting the machine learning models and graph analysis algorithms to find and classify these illegal activities within blockchain transactions in a much easier and simpler way. The researchers also have started planning to extend their studies to expand their reach to other blockchain networks beyond Bitcoin.
By broadening the scope of their research, they aim to build on methodologies that can be applied across a variety of different blockchain platforms, which will end up enhancing the overall effectiveness of forensic analysis in uncovering illegal financial activities that are happening in the cryptocurrency space.
These efforts are so important when it comes to the growing “toughness” of financial crimes in the digital space. The findings from this research show the real potential of blockchain data analysis in efforts to not just fight against, but bring an end to money laundering, fraud, and other financial crimes in the cryptocurrency ecosystem.
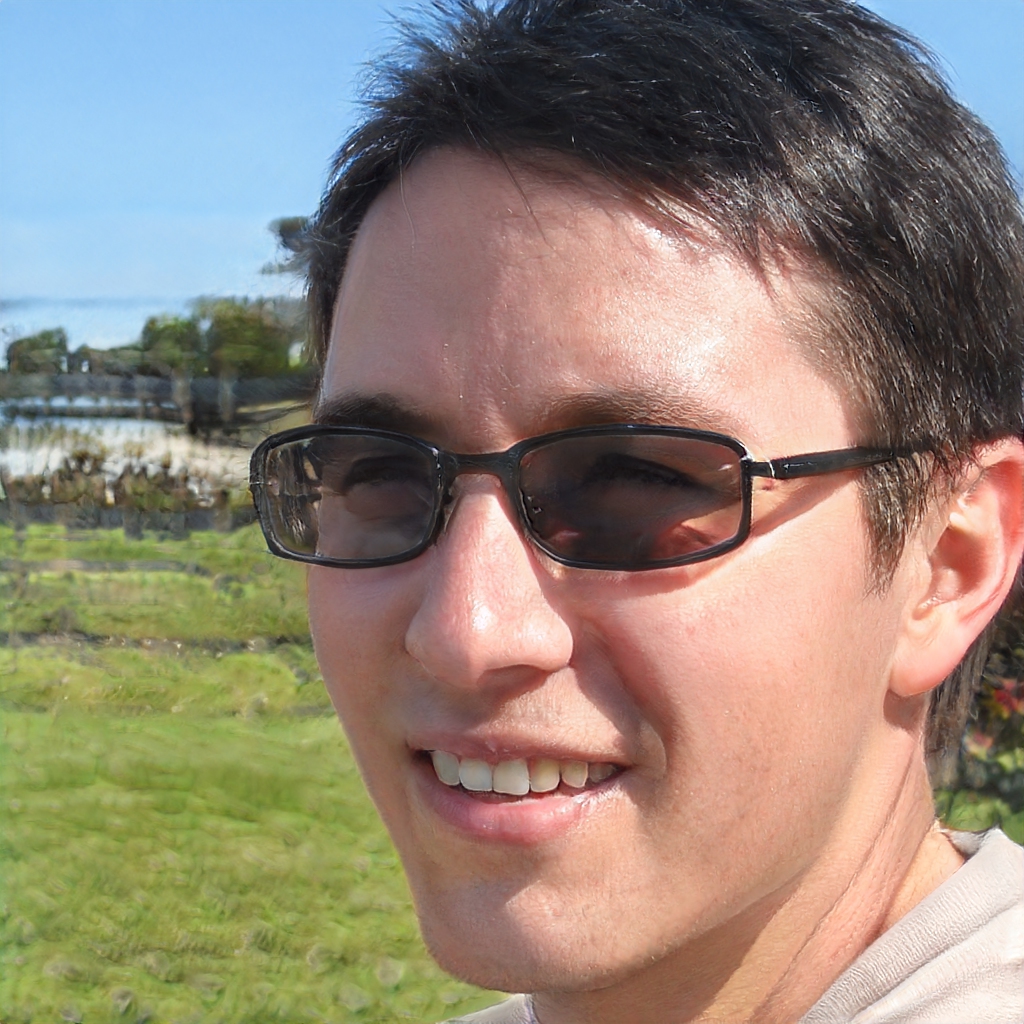
Hey there, I’m a dark web geek who’s been around for the last 8 years. More precisely, I’m livedarknet’s senior content writer who’s been writing about darknet marketplaces, tutorials, and cybersecurity stuff for educational purposes.